New publication in Geographical Analysis
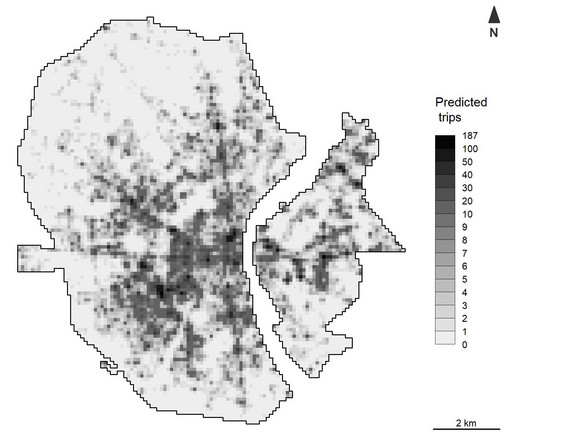
Machine learning methods can offer a useful addition in transport and spatial research: The article published by Katja Schimohr, Philipp Doebler (Faculty of Statistics) and Joachim Scheiner in the current volume of Geographical Analysis deals with the modelling of the spatial distribution of bikesharing trips in Cologne. For this purpose, it compares a generalised additive model (GAM) with the XGBoost algorithm from the field of Machine Learning. In both methods, measures are taken to account for spatial autocorrelation. While the results of the GAM give an easier to interpret insight into the factors influencing bikesharing use, XGBoost leads to more accurate predictions. Both methods are well suited for spatial modelling of demand, although the choice of method should be made depending on the objective of the study.